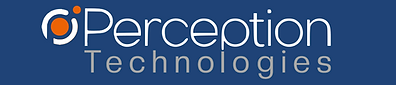
Innovative technology to improve safety
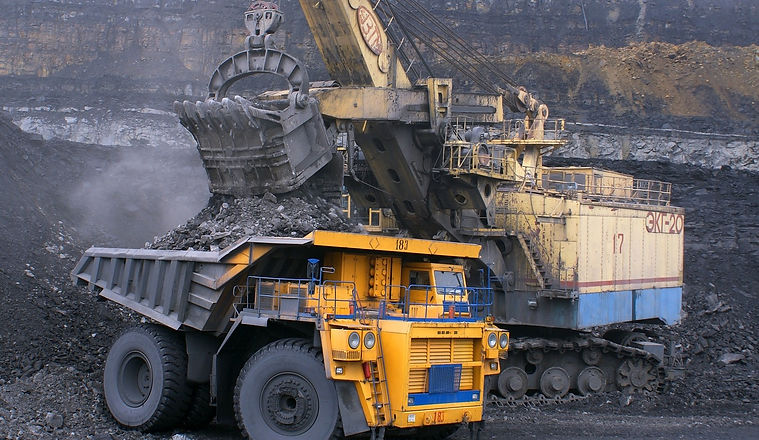
RISK PROPENSITY

Our technology analyzes subtle facial-vocal expressions, and cognitive parameters to identify risk propensity
Data and accuracy
Our algorithms combine the most reliable and innovative technology to detect moods that predispose workers to trigger accidents, either through negligence or cognitive blindness.
Artificial emotional intelligence
Emotion AI is also known as emotion recognition or emotion detection technology, it is commonly referred to as facial coding.
Humans use a lot of non-verbal cues, such as facial expressions, gesture, body language and tone of voice, to communicate their emotions. Our vision is to detect those emotions that blind people to trigger accidents. Our long term goal is to develop “Multimodal Emotion AI”, that combines analysis of both face and speech as complementary signals to provide richer insight into the human expression of emotion. Our combined emotional analysis includes cognitive and personality tests in order to provide the most accurate and innovative service.
Emotion detection – Face
Our Emotion AI unobtrusively measures unfiltered and unbiased facial expressions of emotion, using any optical sensor or just a standard webcam. Our technology first identifies a human face in real time or in an image or video. Computer vision algorithms identify key landmarks on the face – for example, the corners of your eyebrows, the tip of your nose, the corners of your mouth. Deep learning algorithms then analyze pixels in those regions to classify facial expressions. Combinations of these facial expressions are then mapped to emotions.
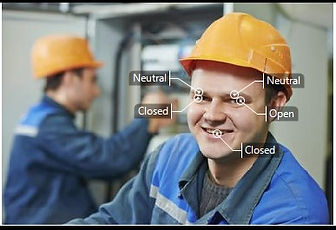
METHODOLOGY
The following list is only a small selection of scientific publications relevant to our work:
Alan S. Cowen and Dacher Keltner
Self-report captures 27 distinct categories of emotion bridged by continuous gradients PNAS 2017 114: E7900-E7909
Arkes, H. R., Herren, L. T., & Isen, A. M. (1988). The role of potential loss in the influence of affect on risk-taking behavior. Organizational Behavior and Human Decision Processes, 42(2), 181-193.
Breaban, A., and Noussair, C. N. (2014). Emotional State and Market Behavior. Working paper, Tilburg University.
Breaban A, van de Kuilen G and Noussair CN (2016) Prudence, Emotional State, Personality, and Cognitive Ability. Front. Psychol. 7:1688. doi: 10.3389/fpsyg.2016.01688
Chentsova-Dutton, Y. E., and Tsai, J. (2010). Self-focused attention and emotional response: the role of culture. J. Pers. Soc. Psychol. 98, 507–519. doi: 10.1037/a0018534
Crivelli, Carlos & Russell, James & Jarillo, Sergio & Fernandez-Dols, José-Miguel. (2016). The fear gasping face as a threat display in a Melanesian society. Proceedings of the National Academy of Sciences. 113. 12403-12407. 10.1073/pnas.1611622113.
Deck, C., and Schlesinger, H. (2010). Exploring higher order risk effects. Rev. Econ. Stud. 77, 1403–1420. doi: 10.1111/j.1467-937X.2010.00605.x
Deck, C., and Schlesinger, H. (2014). Consistency of higher order risk preferences. Econometrica 82, 1913–1943. doi: 10.3982/ECTA11396
Ebert, S., and Wiesen, D. (2011). Testing for prudence and skewness seeking. Manage. Sci. 57, 1334–1349. doi: 10.1287/mnsc.1110.1354
Ebert, S., and Wiesen, D. (2014). Joint measurement of risk aversion, prudence, and temperance. J. Risk Uncertain. 48, 231–252. doi: 10.1007/s11166-014-9193-0
Eckel, C. and Grossman, P. J. (2008). “Men, women, and risk aversion: experimental evidence,” in The Handbook of Experimental Economic Results, eds C. Plott and V. Smith (Amsterdam: Elsevier), 1061–1073.
Eeckhoudt, L., and Schlesinger, H. (2006). Putting risk in its proper place. Am. Econ. Rev. 96, 280–289. doi: 10.1257/000282806776157777
Ekman, P. (2003). Emotions Revealed, 2nd Edn. New York, NY: Times Books.
Ekman, P., and Friesen, W. (2003). Unmasking the Face: A Guide to Recognizing Emotions from Facial Clues. Los Altos, CA: Alor publishers.
Ekman, P., Friesen, W. V., O'Sullivan, M., Chan, A., Diacoyanni-Tarlatzis, I., Heider, K., et al. (1987). Universals and cultural differences in the judgments of facial expressions of emotion. J. Pers. Soc. Psychol. 53, 712.
Henning, Jaime & Stufft, Carolyn & Payne, Stephanie & Bergman, Mindy & Mannan, M. Sam & Keren, Nir. (2009). The influence of individual differences on organizational safety attitudes. Safety Science. 47. 337-345. 10.1016/j.ssci.2008.05.003.
Jeffries, Francis. (2017). Predicting Safety Related Attitudes in the Workplace: The Influence of Moral Maturity and Emotional Intelligence.
Kugler, T., Connolly, T., and Ordonez, L. D. (2012). Emotion, decision, and risk: betting on gambles versus betting on people. J. Behav. Decis. Making 25, 123–145. doi: 10.1002/bdm.724
Lerner, J. S., & Keltner, D. (2001). Fear, anger, and risk. Journal of Personality and Social Psychology , 81 (1), 146-159.
Linares, J. (2003). La concepcion Heideggeriana de la tecnica: Destino y peligro para el hombre. Signos filosoficos, num 10, julio-
Lottridge, Danielle & Chignell, Mark & Jovicic, Aleksandra. (2011). Affective Interaction Understanding, Evaluating, and Designing for Human Emotion. Reviews of Human Factors and Ergonomics. 7. 197-217. 10.1177/1557234X11410385.
Nguyen, Y. and Noussair, C. N. (2014), Risk Aversion and Emotions. Pacific Economic Review, 19: 296–312. doi:10.1111/1468-0106.12067
Effects of specific emotions on subjective judgment, driving performance, and perceived workload
M Jeon, BN Walker, JB Yim
Transportation research part F: traffic psychology and behaviour 24, 197-209
Shichuan Du, Yong Tao, and Aleix M. Martinez. Compound facial expressions of emotion. PNAS 2014 111: E1454-E1462.
Thaler, R., and Johnson, E. (1990). Gambling with the house money and trying to break even: the effect of prior outcomes on risky choice. Manage. Sci. 36, 643–660.
Treich, N. (2010). Risk-aversion and prudence in rent-seeking games. Public Choice 145, 339–349. doi: 10.1007/s11127-009-9569-x
Tversky, A., and Kahneman, D. (1992). Advances in prospect theory: cumulative representation of uncertainty. J. Risk Uncertain. 5, 297–323.
Van Leeuwen, B., Noussair, C. N., Offerman, T. J. S., Suetens, S., Van Veelen, M., and Van de Ven, J. (2014). Predictably Angry: Facial Cues Provide a Credible Signal of Destructive Behavior. Working paper, Tilburg University.
Van Paassen, Marinus M.. (2010). Heidegger versus Carthesian Dualism or where is my hammer?. Conference Proceedings - IEEE International Conference on Systems, Man and Cybernetics. 1684-1688. 10.1109/ICSMC.2010.5642318.
White, L. (2008). Prudence in bargaining: the effect of uncertainty on bargaining outcomes. Games Econ. Behav. 62, 211–231. doi: 10.1016/j.geb.2006.11.006